As machine learning frameworks such as Tensorflow and PyTorch become easier to use and pre-designed models for computer vision and natural language processing become more common and powerful, data scientists face a significant challenge in data collection and processing.
Businesses often struggle to collect large amounts of data within a specific time frame to train accurate models. Manually labeling data is expensive and time-consuming to retrieve data. That’s where they use synthetic data.
What is synthetic data?
Synthetic data is an innovation that can help data scientists and businesses overcome data collection barriers and develop reliable machine-learning models faster.
Synthetic data sets are not constructed from records of actual events but are created by a computer program. The primary purpose of the generated data sets is to provide a generic and robust way to train machine learning models.
It is useful for machine learning classifiers that must have certain properties. Data can be categorical, binary, or numeric, but the dataset must be randomly generated. The random data generation process should be controllable and based on specific statistical distributions. You can also place random noise in your data set.
When using this data in a classification algorithm, you should be able to customize the amount of class separation to make your classification problem easier or more complex, depending on the problem requirements. A regression task, on the other hand, can generate data using a non-linear generation process.
The privacy and security risks of synthetic data
Privacy concerns around user data collection and storage practices are growing. In the 2015 Cambridge Analytica data scandal, millions of users discovered that their data was being collected without their consent. In 2021, LinkedIn received public criticism after leaked user information was sold on the dark web.
The US Federal Privacy Act (1974) restricts the use of sensitive data directly related to an individual’s identity, known as personally identifiable information (PII). This includes social security numbers, phone numbers, and addresses. These laws restrict the collection and disclosure of PII and define specific sectors with strict regulatory requirements, including health (HIPAA) and finance (FCRA).
In recent years, governments worldwide have enacted stricter regulations to protect PII and personal data. Data privacy regulations, from GDPR in Europe to CRPA in California, require businesses to obtain explicit consent before collecting personal data, formalizing users’ right to delete their data, and protecting it from cybercrime. Following most of these regulations ensures the protection of images with biometric identifiers that constitute personal data.
Any organization generating synthetic data must perform a privacy assessment. It is to ensure that the synthesized data generated is not real personal data or too similar to such data. This privacy assurance assesses the extent to which synthetic data can identify a data subject and how much new data about that subject gets disclosed after successful identification.
Expected adverse effects of synthetic data on data protection:
- Output control can be complex—especially for complex data sets. The best way to ensure accurate and consistent output is to compare synthetic data with raw or human-annotated data. However, this comparison requires access to the original data.
- The difficulty of plotting outliers—synthetic data can mimic accurate data. Therefore, some outliers in the original data may not be included in the synthetic data. However, outliers may be more critical than expected data points in some applications.
- Model quality depends on the data source—the quality of synthesized data is similar to the quality of the original data and the model that generates it. The data can reflect biases in the original data. Also, manipulating the data set to create a fair synthetic data set can lead to inaccurate results.
How to mitigate synthetic data security risks
Below are the measures based on our research that you can use to reduce synthetic data security threats:
Data discovery tools
Data discovery tools help identify and locate sensitive data to ensure you effectively secure or remove this data. Organizations access and store massive amounts of data daily, making sensitive data discovery a major challenge even with proper data maintenance and storage. However, organizations that do not know where various types of data are located can encounter critical security issues and find it difficult to mitigate potential risks.
Sensitive data discovery is essential for organizations and industries that comply with strict regulations. Financial services, healthcare institutions, government agencies, and telecommunication companies must adhere to strict sensitive data discovery requirements and follow industry-based regulations.
Here are notable benefits of sensitive data discovery tools:
- Effectively identify and locate sensitive data across known and previously unknown data sources.
- Optimize data storage and security.
- Classify sensitive data.
- Improve the organization’s understanding of the relevant risks and determine how to mitigate these risks.
AIOps
AIOps can help implement large-scale data monitoring and analysis to alert and contextually identify problems in an IT environment efficiently. It can also improve behavioral trend analysis and set up automated remediation for specific cases. Here are key features:
- Data classification and monitoring—AIOps analysis engines use known content types, patterns, and predefined policies to process data uploaded and created in the protected environment, classify and tag data, and monitor for access.
- Fraud detection—financial firms and insurers typically require enormous inputs, data types, and intensive processing to implement fraud detection. This process combines text mining, social network analysis, anomaly detection, database searches, and predictive models. AIOps help make this process more efficient.
- Endpoint and network behavior modeling—modeling endpoint communications and behavior patterns can help detect subtle indicators of attack or compromise before any significant data breach or access can occur.
- Threat intelligence analysis—AIOps employs various internal IT operational data and threat intelligence from external providers to predict or circumvent attacks targeting cloud infrastructure, such as account hijacking.
Application governance
Application governance includes the policies and rules businesses use to manage their applications. It aims to increase data security, manage risk, and keep applications running smoothly.
Application governance helps development teams better plan and manage all aspects of an application, including how assets are deployed, how systems are integrated, and how data is protected.
These programs ensure complex application environments meet an organization’s security policies, best practices, and compliance requirements.
Data privacy management
Data privacy management enables organizations to protect sensitive data and remediate privacy breaches. These tools assess the impact of technological changes on privacy, align IT activities with privacy regulations, and track events that may lead to unauthorized disclosure of personal data.
The software helps organizations secure sensitive data in large, distributed environments, automate processes and policies to improve scalability and efficiency and reduce human error when complying with regulations and standards.
This type of solution usually provides the following features:
- Data classification—Automatically analyzes data patterns to identify personally identifiable information (PII) and determine sensitivity levels for security and compliance. This is also useful for reclassifying sensitive data when compliance requirements change.
- Data privacy regulation compliance—ensures personal data meets compliance requirements, provides auditing capabilities to compliance agencies, and identifies compliance risks.
- Remediation—provides remedial guidance on data privacy risks. This allows teams to prioritize and address privacy issues before they lead to security issues or compliance violations.
Share this article
About the Author

Hasnain Khalid is a passionate streaming and security enthusiast, who has proved his expertise on renowned tech publishers. With a keen eye for online safety and a love for all strеaming matters, Hasnain combinеs his еxpеrtisе to navigatе thе digital world with confidеncе and providе valuablе insights to usеrs worldwidе.
More from Hasnain KhalidRelated Posts

19 Best Vulnerability Management Software or Tools in 2024
KEY TAKEAWAYS Vulnerability management tools scan and detect weaknesses within the network that hac...

How to Detect, Identify and Fix Packet Loss with Best Tools
KEY TAKEAWAYS Packet loss reduces the speed and amount of data that flows through the network. This ...
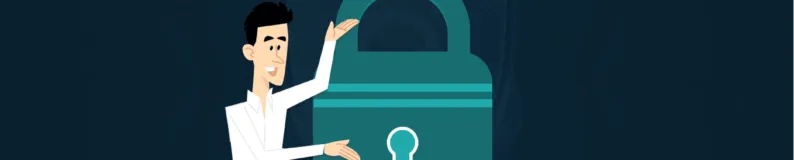
15 Best Network Security Software – Top Pick Of Organizations
KEY TAKEAWAYS Network security software keeps the data secure and blocks malicious or potentially vu...
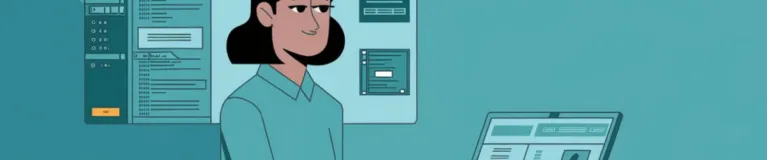
15 Best Virtual Machine Software for Windows in 2024
KEY TAKEAWAYS Virtual machine software is a vital tool for developers to deploy VM software to test ...
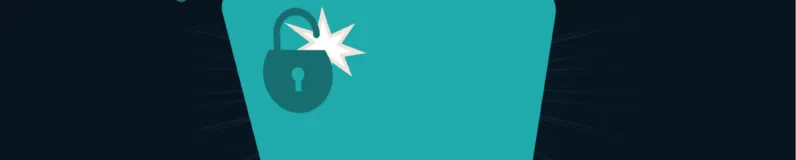
What is Software Deployment: Risks and Best Practices
KEY TAKEAWAYS Software deployment is facing various security risks amidst the advancements in the in...
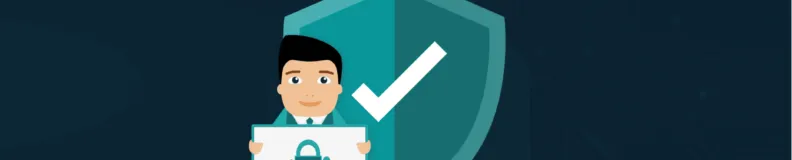
Building Encryption into the Network Fabric with SASE
A network fabric is a mesh of connections between network devices such as access points, switches, a...